Functions & Automation
Welcome to Week 6!
Welcome to week 6 of Earth Analytics! This week you will learn about efficient coding practices. Specifically, you will learn how to use functions to make our code:
- Simpler and easier to read.
- More modular.
- More efficient.
You will also learn the DRY principle of programming - Don’t Repeat Yourself.
Time | Topic | Speaker |
---|---|---|
9:30 - 9:45 | Questions / Review | |
9:45 - 10:15 | Write efficient, expressive code - Don’t Repeat yourself DRY | |
10:15 - 11:00 | Write functions in R | |
11:- 12:20 | Loops & functions to automate workflows |
1a. Readings
There are no new readings for this week.
2. Complete the Assignment Below (5 points)
Homework Submission
Produce a R Markdown Report
Create a new R markdown
document. Name it: lastName-firstInitial-week6.Rmd Within your .Rmd
document, include the plots listed below. When you are done with your report, use knitr
to convert it to html
format. Submit both the .Rmd
file and the .html
(or .pdf
file to D2L. Be sure to name your files as instructed above!
Use knitr Code Chunk Arguments
For this week’s assignment please do not hide your code. We will grade the assignment based upon your use of functions and for loops to complete your assignment.
Answer the Following Questions Below in Your Report
- Define the acronym DRY. What does DRY mean?
- When you document a function, what elements should you include?
- Provide an example of a function name that is expressive vs one that is not expressive.
- Explain the key difference between a variable that you create when programming line by line compared to a variable that is created within a function. Use the example below to help you answer the question OR use code to answer the question.
# this code below should help you answer question 3.
my_variable <- 1+2
# and a variable created within a function
the_answer <- function(num1, num2){
# calculate sum
my_variable2 <- num1 + num2
return(my_variable)
}
# given the code above, will the code below run or return an error? Why?
`my_variable2`
Code Assignment
PART ONE:
For this week’s assignment, write some code that does the following.
- Write a
for loop
that breaks up the file:"data/week-02/precipitation/805325-precip-daily-2003-2013.csv"
into yearly.csv
files. - Each
.csv
file should be saved indata/week-06/
. - Each
.csv
file should contain amonth
column with the month specified as a numeric value between 1-12.
# read in the data - but be sure to address na values when you read it in here!
boulder_precip <- read.csv("path-here-dont-forget-na-arguments")
# fix the date using dplyr pipes
# define min and max year
# build your loop
for (the_year in min_yr:max_yr) {
# use pipes to filter the data by year
# export a .csv file with the year in the name to your data/week-06/ dir
}
HINT: If you followed along in class, then you have already written this code! This lesson will help you complete this task.
PART TWO:
For each of the .csv
files that you created above:
- Create a new
.csv
file that contains the total monthly precipitation in mm. - Name that file
data/week-06/outputs/precip_mm/precip-year.csv
- note that you will need to make new directories to save the file to the path listed.
An example of what the final data should look like is below:
# open data
precip_2003 <- read.csv("data/week-06/outputs/precip_mm/precip-2003.csv")
head(precip_2003, n = 6)
Use functions to complete this task as follows:
- Create a function called
check_create_dir()
that takes a path to a directory that you want to make and checks to see if it exists and then creates it if it doesn’t exist. - Create a function called
in_to_mm()
that converts values in inches to mm (you did this in an earlier lesson so you may already have this function in your code).
NOTES:
- Be sure to consider
NA
values in your data (e.g. 999.99) when you read your data! - Make sure you address
NA
values when you run thesum()
function. - When you write the
.csv
make sure that you addressNA
values!
check_create_dir <- function(dir_path){
# document your function here
# include the code required to check for the directory and then create it here
# because this function is just creating a directory, you don't need to return anything!
}
in_to_mm <- function(precip_in){
# document your function here
# include the code required to convert inches to mm here
return(precip_mm)
}
# create an object with the directory name
new_dir <- "data/week-06/outputs/precip_mm/"
# check to see if the directory exists - make it if it doesn't
check_create_dir(new_dir)
# print the name of each file
for (file in all_precip_files) {
# read in the csv - be sure to fill in the na strings argument - i didn't do that below
the_data <- read.csv(file, header = TRUE, na.strings = 999.99) %>%
mutate(precip_mm = in_to_mm(HPCP)) # add a column with precip in mm and a column with just the month using the month() function
# group the data by month
# summarise using the sum function - be sure you address na values when you sum! we discussed this during week 1
# write output to a new .csv file
write_csv(the_data, path = paste0("data/week-06/outputs/precip_mm/", basename(file)))
}
Bonus Opportunity - 1 point
Use the lapply()
function (instead of a for loop) to
- Process all of the precipitation data files files that you created for each year.
- Add a new column to the file containing anything that you’d like.
- Write a new
.csv
file to a new directory with that output file.
You can chose to use the same code that you used for the homework assignment, however implemented in a for loop if you want.
Homework Due: Monday October 16 2017 @ 8AM.
Submit your report in both .Rmd
and .html
format to the D2L dropbox.
Grade Rubric
R Markdown Report Structure & Code: 10%
Full Credit | No Credit |
---|---|
html / pdf and RMD submitted | |
Code is written using “clean” code practices following the Hadley Wickham style guide | |
Code chunk contains code and runs | |
All required R packages are listed at the top of the document in a code chunk | |
Code chunk arguments are used to hide warnings & messages | |
All code is visible in the knitted document | |
Lines of code are broken up at commas to make the code more readable |
Report Questions: 30%
Full Credit | No Credit |
---|---|
Define the acronym D.R.Y.. What does DRY mean? | |
When you document a function, what documentation elements should you include? | |
Provide an example of a function name that is expressive vs. one that is not expressive | |
Explain the key difference between a variable that you create when programming line by line compared to a variable that is created within a function |
Code is Worth 60% of the Assignment Grade This Week
For Loop 1 & General for Loop 2
Write a loop that takes the file “data/week-02/precipitation/805325-precip-daily-2003-2013.csv” and creates an individual
.csv
file for each years worth of data.
Full Credit | No Credit |
---|---|
Code produces an individual .csv file for each year’s worth of data | |
Following the code, .csv files are saved in the data/week-06/ directory | |
.csv files are named correctly - including the year of data that the file contains | |
NA values are handled properly in the code - when the data are read in, and exported to .csv files and for the monthly summary calculation | |
.csv files created contain the correct data (for the year specified) |
Specific for Loop 2
Loop through the files that you created in part one and summarize the data by monthly total precipitation in inches. Create new
.csv
files for each year.
Full Credit | No Credit |
---|---|
in_to_mm() function is used to convert precipitation from inches to mm | |
check_create_dir() function is used to check for and create a directory if one doesn’t exist | |
Data in individual yearly .csv files are summarized by month | |
.csv files are saved in the data/week-06/outputs/precip_mm/ directory | |
All functions are documented with what the function does, inputs, outputs and structure of inputs and outputs |
Share on
Twitter Facebook Google+ LinkedIn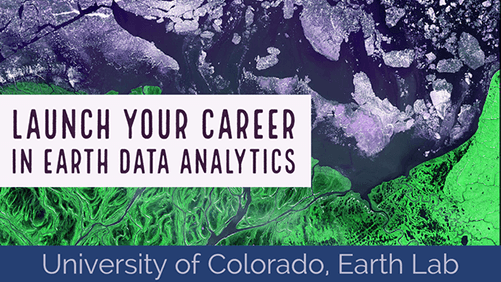